Radiomics and quantitative image analysis
Radiomics refers to a type of analysis of medical images in which a large number of quantitative image features, e.g. of a tumour, are extracted using computer algorithms. Radiomics allow capturing the appearance of tumours on images quantitatively and in greater detail than in routine radiological reporting.
​
In a Radiomics analysis pipeline the first step of image processing is often the outlining of the diseased structures, such as a tumour, on the medical images (= segmentation; creation of so-called regions of interest; ROIs).
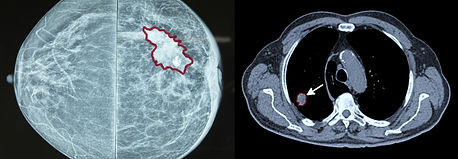
Radiomics
Afterwards, especially developed computer programmes allow the calculation of Radiomics features, which primarily describe tumour size, shape and texture with the help of numbers.
Statistical methods and machine learning techniques are then used to select the most meaningful features and to make clinically meaningful predictions based on the image material (e.g. treatment response, prognosis, tumour subtype, tumour-specific gene mutations, etc.).
Imaging
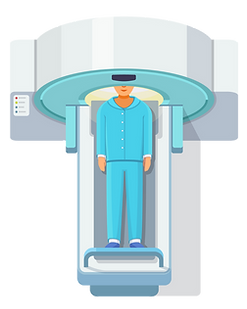
(A)
Segmentation
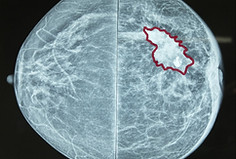
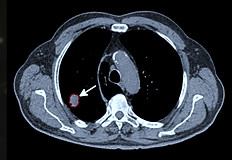
(B)
Radiomics
Tumour Size & Shape
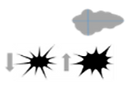
Volume
Diameter along different​
​axes
Compactness
Frequency & spatial distribution of grey levels

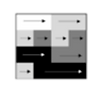
Texture Analysis
GLCM (Grey level co-occurrence matrix)
Histogram Analysis
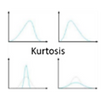
Skewness
Contrast=

Entropy=

(C)
AI
for the prediction of Clinically
meaningful variables
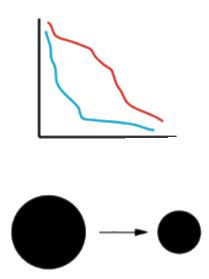
Survival
Tumour Size
(D)
Illustration of a radiomics pipeline using breast cancer imaging as an example. After the medical images are taken.
​
​
(A) the tumour is manually marked on MRI images (segmentation = creation of regions of interest, ROIs;
​
(B) Radiomics features are then calculated according to predefined formulas
(C) for example, the tumour volume and various shape features such as diameter along different axes, compactness, sphericity (similarity to spherical shape) etc. are calculated. Based on the pixels and their grey levels within the tumour, histogram analysis parameters are calculated based on their frequency distribution and texture features are calculated considering the spatial distribution and neighbourhoods of the pixels (such as the features of the so-called grey level co-occurrence matrix).
​
(D) Finally, statistical methods and AI techniques are used to reduce the number of relevant radiomics features and to develop predictors from the remaining radiomics for predicting, for example, patient prognosis or treatment response.